Advancing Electric Vehicle Battery Management: How Will Science-Based AI Contribute
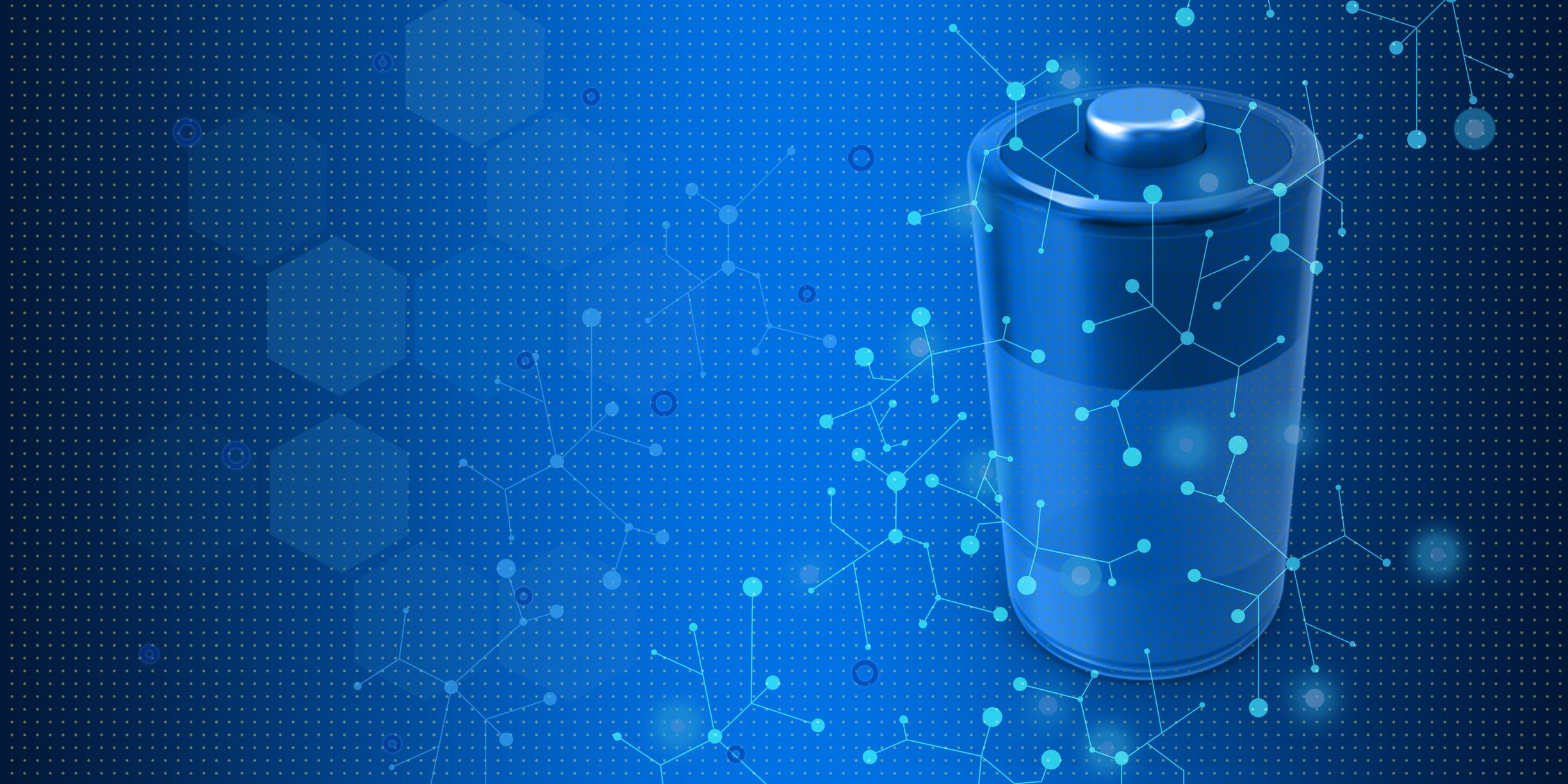
Due to climate change and the influence of greenhouse gas emissions (CO2 in particular), use of fossil fuels to power vehicles is being aggressively curtailed globally. Government intervention in favor of electric powertrains in the form of subsidies and carbon taxation methods is pushing increased production of battery-powered vehicles. The hope is that public adoption will follow, and that charging will be increasingly done via renewable sources of electricity. All things combined, a significant reduction in net carbon emissions from transportation systems is anticipated in the coming decade, which should have a favorable impact on climate trends.
Although a plethora of battery technologies are available, lithium-ion batteries are currently dominating the electric vehicle industry as both primary (full electric systems) and secondary (hybrid gas-electric) power sources. It is anticipated that lithium-metal batteries may gain increased market share in the future, with the main difference being increased capacity and energy density due to use of lithium metal anodes (vs. most commonly graphite or similar materials) with a concomitant increase in required safety features because of the hazards of lithium metal. Regardless of the type of lithium battery (ion or metal), a complex battery management system (BMS) is required to properly monitor, control, and optimize the performance of one or more battery modules.1,2
What Constitutes a Battery Management System?
The main functions of an electric vehicle BMS are to monitor and regulate the charging, discharging, temperature, safety, fault diagnosis, and other key operating parameters to maintain adequate performance and safe vehicle operation. The BMS consists of a series of hardware systems (circuit board, chips, sensors, etc.) and related control software. The operating system is typically required to function in real-time (RTOS) to allow rapid response to changing conditions.
Battery management systems offer many benefits that are primarily focused on improved performance and safety management. Battery cell, module, and/or pack monitoring allows the system to gain valuable data about current and voltage levels to aid the protection systems and allow additional operational decisions. Optimal charging and discharging helps to maintain battery performance and durability, and the BMS is critically important to achieve proper charge/discharge conditions (e.g., constant current-constant voltage charging; discontinuous current mode discharge).
Another key aspect of the BMS is the estimation of battery states (charge, health, and function). There are many mathematical systems used for generating these estimates from measured parameters and look-up tables (or similar systems) based upon prior test results under controlled conditions. The state estimations allow communication of remaining range, overall battery health, and possible maintenance requirements.1 Equally important functions of the BMS are centered around protecting the battery from thermal runaway via temperature management, imbalanced charge and voltage between cells, overcharging, undercharging, as well as detecting possible faults and communicating an appropriate action plan. To do all of this, a tremendous amount of data acquisition, storage and transfer is required.

Shortcomings With Existing Battery Management Systems
Given that there are a wide variety of models and algorithms being used for state estimation, aging effects, and fault detection, there is no consensus standard approach. Indeed, the accuracy of many of the existing approaches could be improved. Also, many battery management systems in use today do not collect complete current, voltage, and temperature data at the cell level nor do systems adequately utilize the data being collected to provide real-time feedback beyond use of look-up tables that may not adequately account for actual operating conditions (e.g., temperature, load/power demand) and the individual battery cell, module, and pack history. Given the large number of possible failure conditions and the strong influence of charge, discharge, and temperature history on future performance, collection of higher quality data and more effective real-time use of it may be necessary to move battery management to the next level of range performance, cell balancing, and safety optimization.
Can AI and Machine Learning Methods Contribute?
With a potentially very large volume of data being collected before an electric vehicle is released (e.g., experimental testing to create look-up tables) as well as during operation from individual vehicles and full fleets, it is intuitive that AI and machine learning approaches that are well suited for analyzing and modeling large data sets should be utilized as part of the BMS. One major hurdle with such implementation is the lack of publicly available data for model development and testing. Additionally, there are large performance profile differences between battery configurations and even within identical battery systems with different operating histories. Therefore, each electric vehicle BMS research and development group tends to operate in isolation and those lacking adequate AI and machine learning expertise may not fully realize the benefits without engaging with external partners. There are also barriers to entry that must be overcome within the automotive industry when it comes to adoption of new technologies.
Despite those issues, there have been several attempts to integrate machine learning approaches into the BMS, primarily with regard to state estimations (state of health, state of charge, and remaining useful life), cell management, and fault diagnosis/prognosis, although few, if any, have been implemented in commercial electric vehicle systems.,,, Various machine learning techniques have been applied to these BMS problems, including Supervised Learning via artificial neural networks and support vector machines, Unsupervised Learning, and Reinforcement Learning, with each having multiple sub-categories of algorithm types.3 Here at NobleAI, we feel that there are significant gains to be realized via use of AI and machine learning methods in the BMS development process and that the time to do this is upon us.
NobleAI
Distinct from conventional AI models that lack scientific interpretability, NobleAI utilizes a science-based AI (SBAI) approach that integrates data-driven methodologies with scientific principles, ensuring that AI-driven predictions are bounded by real-world considerations. We leverage our in-house expertise and your specific domain knowledge to identify appropriate scientific principles to apply to the problem at hand. Additionally, NobleAI utilizes advanced data processing and filtering techniques that allow use of imperfect real-world data (e.g., noisy, sparse) in our machine learning models. This multi-faceted approach allows for comprehensive problem-solving, extrapolation beyond training data constraints, and predictions that are rooted in scientific knowledge. The result is enhanced precision, transparency, and reliability when it comes to battery performance modeling. Models for accelerated testing, state of charge, state of health, end of useful life, condition-based charge/discharge effects, fault diagnosis, and real-time data integration are potential areas for collaboration. Bring your electric vehicle BMS challenges forward and we will work with you to find science-based AI solutions.
_____________________________________________________________
1 Hannan, M.A. et. Al., “State-of-the-Art Energy Management System of Lithium-ion Batteries in Electric Vehicle Applications: Issues and Recommendations” IEEE Access2018, 6, 19362.
2 Gabbar, H.A. et. Al., “Review of Battery Management Systems (BMS) Development and Industrial Standards” Technologies2021, 9, 28.
3 Ardeshiri, R. R. et. Al., “Machine Learning Approaches in Battery Management Systems: State of the Art: Remaining useful life and fault detection” IEEE Xplore 2020, 61.
4 Onori, S. et. al., “Analysis and key findings from real-world electric vehicle field data” Joule2023, 7, 1.
5 Harippriya, S. et. al., “Battery Management System to Estimate Battery Aging Using Deep Learning and Machine Learning Algorithms” J. Phys. Conf. Series, 2022, 2325, 012004.
6 Ghosh, A. et. al., “State of Charge Estimation of Lithium-ion Battery for Electric Vehicles Using Machine Learning Algorithms” World Electric Vehicle Journal, 2021, 12, 38.
7 Abdullah, H. M., et. al., “Reinforcement Learning Based EV Charging Management Systems-A Review” IEEE Access2021, 9, 41506.
8 Duraisamy, T. et. al., “Machine Learning-Based Optimal Cell Balancing Mechanism for Electric Vehicle Battery Management System” IEEE Access, 2021, 9, 132846.