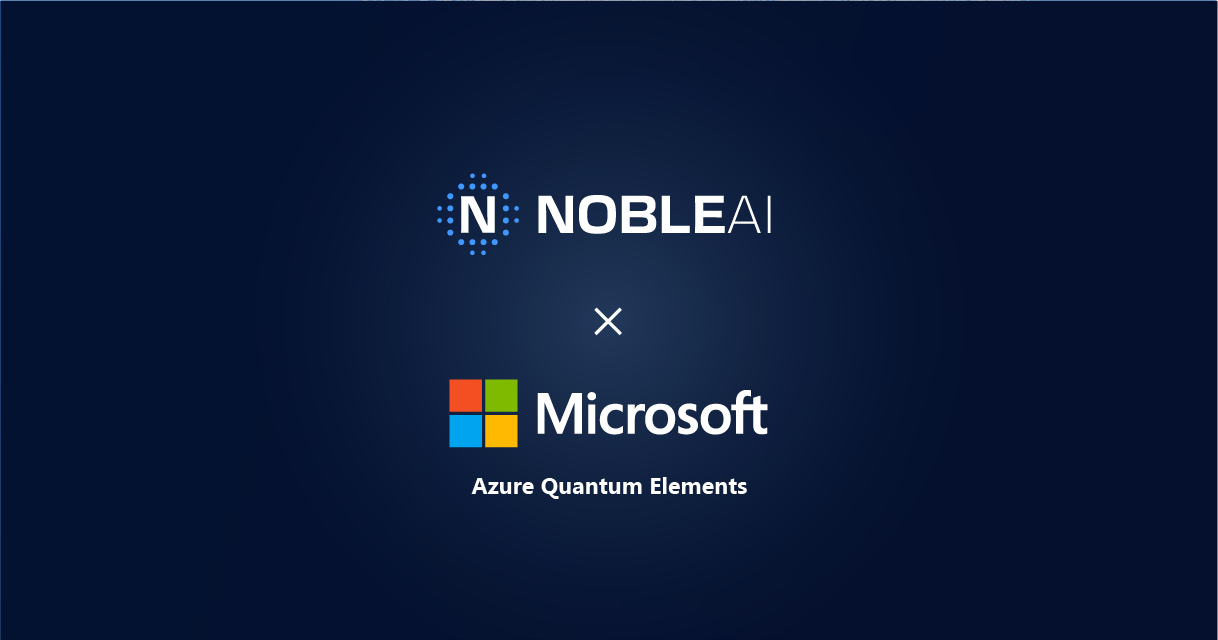
In the ever-evolving landscape of scientific discovery, the power of computation has become a driving force behind groundbreaking advancements. Over the centuries, we have witnessed a systematic progression in our computational toolbox, from advanced mathematical and theoretical techniques to physics-based simulations and, more recently, data-driven machine-learning approaches. This progression has opened doors to more sophisticated scientific exploration, making the once impossible, possible.
We are excited for our game-changing collaboration between NobleAI and Microsoft's Azure Quantum Elements (AQE). This partnership pushes forward an emerging “fifth paradigm of science” that builds upon and integrates the best of empirical, theoretical, simulation, and big data approaches to accelerate science. As outlined by Dr. Nathan Baker, Product Manager of Azure Quantum Elements, we want to “compress 250 years of chemistry into the next 25.” Our collaboration will democratize the field of computational chemistry, by uniting the scale offered by AQE with NobleAI’s expertise in building science into AI models for product development.
The Evolution of Computational Science
Chemistry simulations are highly effective, but work best within their domains of validity: the resolutions, length scales, and time scales that they were designed to describe. Scientists now routinely leverage simulations to study catalysis [1], battery cathodes [2], molecular self assembly [3], and much more.
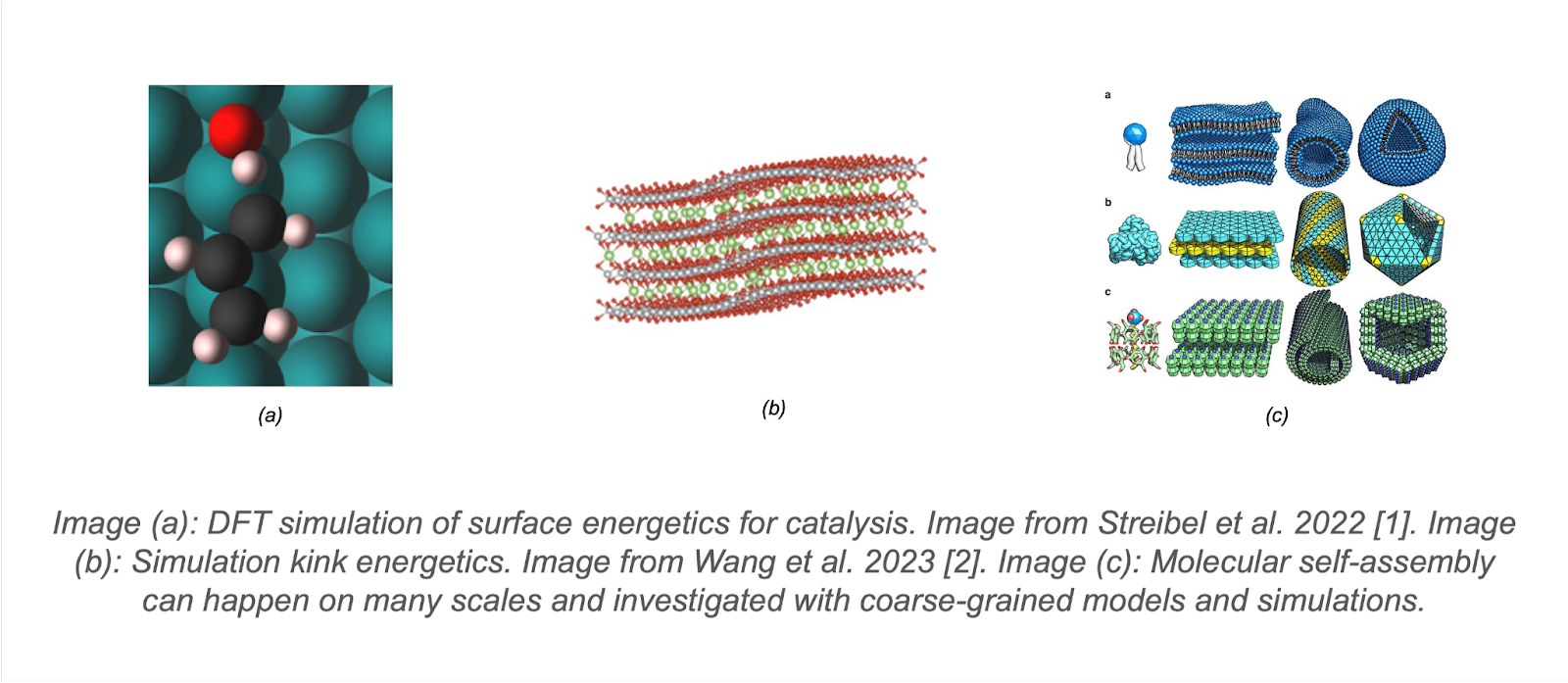
Despite the promise of computational chemistry, two significant hurdles limit its widespread adoption. First, highly specialized knowledge is required both to run computational chemistry simulations and maintain the supercomputing resources required to run the simulations. Secondly, the multi-scale nature of most materials science problems, from atomic arrangements to performance metrics, means that there is a lot of ground to cover.
Our collaboration with Microsoft AQE provides customers with a comprehensive toolbox to overcome these two hurdles and tackle their scientific challenges. Here's how our joint efforts enhance the reach and impact of computational chemistry:
- Democratizing Computational Chemistry: By leveraging AQE, customers gain access to flexible computational chemistry computing capacity without the administrative overhead. Noble AI uses its domain experience to set up proper simulation workflows on the AQE service. This democratizes the availability of computational chemistry, bringing it to a broader audience, and allows scientists to spend more time thinking about science.
- Integrative Machine Learning: NobleAI brings its experience in product-based, Science-Based AI to the table. We connect the scales accessible by simulations to real-life product-scale performance properties crucial for materials development.
- Enhanced Scientific Fidelity: Integrating computational chemistry into machine learning models enhances the scientific fidelity of these models. This means more accurate predictions and deeper insights to accelerate your product development.
So What Is Possible?
In our press release about this new collaboration with Microsoft AQE, we highlighted the opportunities for accelerating time to discovery and innovation. But how does one get to that point? Ever see a cool hybrid simulation-machine learning study and wonder, “what if I could do that for my system and product?” If your company doesn’t have a computational chemistry team, or your computational chemistry team is strapped for time, we can help. To get the creative juices going, here are some potential ideas that we are excited by:
- Find new simulation-driven insights. Chemistry simulations are like microscopes. Have you ever wondered what the conformations of molecules in your system are? How they interact with each other? Or perhaps you wanted to know how chemical functionalization modifies the solubility of your molecules? Or how crystal structure might change the active site of your catalyst? With quantum and classical molecular dynamics simulations, you can get snapshots of molecules in action. Correlate material performance with atomic and molecular structure.
- Derive better machine learning features. Not only can simulations give you nanoscopic insights into your systems, but you can actually leverage simulation data on molecules to derive new, quantitative molecular features for describing your system. These quantitative features can in turn enhance machine learning models, making them both more accurate and transparent. Quantify and operationalize your insights. Use NobleAI’s expanding molecular descriptor (example simulation-derived features: sigma profiles, charge clouds, radial distributions, etc.) toolkit, bring your own, or let us design bespoke features together.
- Accelerate exploration with fast proxy machine learning models. Studying a reaction via Kinetic Monte Carlo? Or catalysis for polymerization? Building fast proxy models of high fidelity computer simulations is a common strategy for using ML to unlock science. However, historically it is often too expensive to run simulations at the scales required to build meaningful data sets. With AQE, NobleAI will now scale computational chemistry calculations so that customers can generate customized datasets to address their particular needs. With a fast proxy model in hand you can then do all sorts of scenario analyses and push the limits of knowledge.
Working Together
NobleAI will work closely with your team to develop the appropriate simulation strategy to complement machine learning in a unified solution.
- Define the problem you would like to understand and solve. What data is available? What properties would you like to target?
- Data. What kinds of data are available? Collect any characterization or materials specifications data that may help describe the material system.
- Simulate. What kinds of simulations do we need to fill in the gaps in knowledge? Simulations come in many scales, shapes, and sizes, each designed to address specific questions. This can be DFT to get at electronic properties of inorganic materials to molecular dynamics to get at molecular conformations to reaction kinetics to follow polymerization processes. These simulations allow us to infuse more science into the ML model – for example, they can be used to generate data for inputs to models, output data for training models, or provide constraints and inspiration for model architecture. Additionally, simulations allow for scientific insights into the underlying physical and chemical processes.
- Scale simulations with AQE to cover more chemistries, generate large data, and develop a more complete picture of the problem.
- Combine NobleAI featurization and model architectures with AQE and any customer characterization data to develop more informative machine-learned models. For speed (e.g. for a high throughput campaign) we would develop emulator (“proxy”) ML models to rapidly predict and approximate the outputs of simulations.[4,5] In certain simulations where time-costs of simulation are acceptable, we can also call AQE “on demand” to generate supplemental data. Lastly, machine learning models could be used to process the outputs of simulation data and identify hidden patterns in the way atoms and molecules interact.[6]
- Deploy science-based models supported by AQE for the prediction of properties. Rapidly explore your chemistry, formulation, and process design space, generate insights, and launch commercial products.
The Future is Now
We are enthusiastic about the possibilities this collaboration will unveil for materials discovery and innovation. Interested in brainstorming ideas? Reach out to us at info@noble.ai.
_____________________________________________________________________
[1] Streibel V, Aljama HA, Yang AC, Choksi TS, Sánchez-Carrera RS, Schäfer A, Li Y, Cargnello M, Abild-Pedersen F. Microkinetic modeling of propene combustion on a stepped, metallic palladium surface and the importance of oxygen coverage. ACS Catalysis. 2022 Jan 18;12(3):1742-57.
[2] Wang C, Wang X, Zou P, Zhang R, Wang S, Song B, Low KB, Xin HL. Direct observation of chemomechanical stress-induced phase transformation in high-Ni layered cathodes for lithium-ion batteries. Matter. 2023 Apr 5;6(4):1265-77.
[3] Frederix PW, Patmanidis I, Marrink SJ. Molecular simulations of self-assembling bio-inspired supramolecular systems and their connection to experiments. Chemical Society Reviews. 2018;47(10):3470-89.
[6] https://www.microsoft.com/en-us/research/project/graphormer/